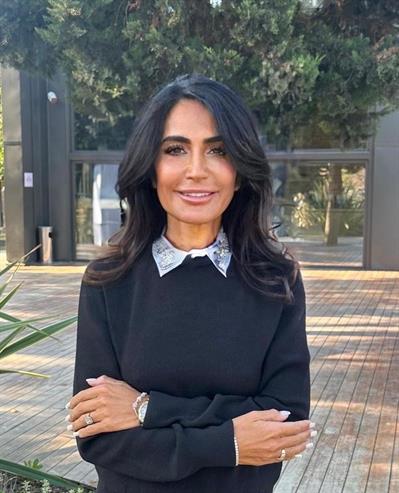
Prof. Yeliz Karaca
University of Massachusetts Chan Medical School (UMASS), USA
Speech Title: Mathematical Modeling, Stochastic Processes and Computational Complexity in Precision Medicine: Medical and Clinical-related Applications with Fractional Calculus Operators, Bloch Torrey PDE, Hidden Markov and AI
Abstract:
Mathematical models are indispensable tools for understanding and simulating complex dynamical systems, particularly in the mathematical models concerning uncertain phenomena are viable for the sake of description and for computation of complex dynamical behaviors, which enable the applicable mathematical formulations pertaining to neural dynamics across the temporal and spatial scales. Herewith, the form of equations facilitating the comprehension of the subtleties of the original problem subsequently also aid in terms of discovering new attributes towards complex problems. Human brain, as one of the most complex networks of nature, displays chaotic, stochastic as well as dynamic aspects inherent in its analysis considering its intricate neurological configuration. Apart from these aspects, human brain owing to its fractal structure manifests complex dynamics with fractals in the brain characterized by diverse characteristics some of which are singularity, irregularity and self-similarity in terms of forms at different observation levels, which could pose challenges in detection since observations in real-time occurrences can prove to be discrete, continuous, noisy or time-variant. Stochastic models, notwithstanding, are characterized in applications where probabilistic observations show functions of the state, constitutions a doubly stochastic process ensuring underlying stochastic analyses and processes’ inferring. Notably, stochastic processes are mathematical models constructed through a family of random variables. Thus, mathematical models are known to provide abstractions through reducing the problem to its fundamental characteristics while addressing the relationship with the flow of interacting elements. In view of these considerations, Hidden Markov Model (HMM), as a stochastic process, posits that latent or implicit stochastic processes can be inferred indirectly by means a sequence of observed states considering that observations are probabilistic functions of the state. Henceforth, HMM is a beneficial for purposes of modeling, simulations as well as applications within a multitude of scientific disciplines. Computational complexity concerning the models are calculated in order that the complexity of equations can be measured relying on the degrees to reduce time parameter and space parameter, related with storage, to enhance compatibility with models ultimately. Furthermore, advanced mathematical models employ multifaceted methods to extract information from the images. Therefore, Diffusion Magnetic Resonance Imaging (DMRI), as an experimental and noninvasive imaging technique, provides viable and effective applications in clinical and medical research, lending an accurate pedigree pertaining to the diffusion characteristics of water in biological tissues. With respect to neuronal dynamics, the afore-cited techniques can provide a reliable way for detecting and generating accurate quantitative parametric instruments to reflect the complex, subtle and intricate properties of brain tissues, which requires one to focus on the precise connections between tissue microstructure and signals. These aspects are critical for precision medicine where individualized conditions and attributes need to be taken into account for diagnostic, prognostic, follow-up processes, prediction of the course of the disease to take it under control, and so forth. All these points need to be meticulously designed and constructed for each patients in a personalized way to enhance and maintain their life quality. For the evaluation of computational complexity pertaining to the finite difference method, Bloch–Torrey partial differential equation and fractional methods manifest the significance of applicable mathematical models for attaining an optimal level of accuracy to identify the total simulation time as well as the diffusion coefficient of the simulated tissue. Accordingly, the critical manifolds of multifaceted mathematical modeling in alignment with Artificial Intelligence (AI) techniques as well as machine learning methods can significantly contribute to robust and accurate scientific understanding and interpretation through the validation of related multidisciplinary aspects and customized needs of the individuals to improve hyperparameter optimization based on complex data-based problems from the viewpoint of solution-oriented systems as well as schemes.
Keywords: Mathematical Neuroscience; Stochastic Processes; Hidden Markov Model (HMM); Markovian processes; Bloch Torrey Partial Differential Equation; Fractional Calculus; Medical Imaging; Multifractal Analysis; Diffusion Magnetic Resonance Imaging (DMRI); Artificial Intelligence (AI); Machine Learning; Computational Complexity; Computational Neuroscience; Neural Dynamics, Accurate Neuron Geometry Models; Mathematical Modeling; Optimal Predictive Dimension of Changes; Hyperparameter Optimization; Precision Medicine.
References:
[1] Karaca, Y., Baleanu, D., & Karabudak, R. (2022). Hidden markov model and multifractal method-based predictive quantization complexity models vis-á-vis the differential prognosis and differentiation of multiple sclerosis’ subgroups. Knowledge-Based Systems, 246, 108694.
[2] Karaca, Y. (2022). Theory of complexity, origin and complex systems. In Multi-Chaos, Fractal and Multi-fractional Artificial Intelligence of Different Complex Systems (pp. 9-20). Academic Press.
[3] Karaca, Y. (2023). Fractional Calculus Operators–Bloch–Torrey Partial Differential Equation–Artificial Neural Networks–Computational Complexity Modeling of The Micro–Macrostructural Brain Tissues with Diffusion MRI Signal Processing and Neuronal Multi-Components. Fractals, 2340204.
Biography:
Yeliz Karaca is an Associate Professor of Applied Mathematics and a Researcher Professor at the University of Massachusetts Chan Medical School (UMASS), USA.
She received her Ph.D. degree in Mathematics in 2012. Along with the other awards she has been conferred, she was granted the Cooperation in Neurological Sciences and Support Award by Turkish Neurology Association as the first mathematician in Türkiye. Besides these, she holds a medical card, based on the long-term various medical and clinical-related trainings she has received and been certified, as the only mathematician entitled to it. Furthermore, she received the Outstanding Young Scientist Award in 2012 and Best Paper Awards in her specialized discipline, among the other national and international awards in different categories as well as grants. Another award of hers is Outstanding Reviewer Award (Mathematics Journal, MDPI) in 2021.
Dr. Karaca is the Editor-in-Chief of the book series named Systems Science & Nonlinear Intelligence Dynamics by World Scientific, and she has been acting as the lead editor, editor and associate editor in many different SCI indexed journals. She has books some of which are Computational Methods for Data Analysis published in 2018 by De Gruyter, an edited book named Computational Science and Its Applications – Lecture Notes in Computer Science (ICCSA 2023, with series 1-9) by Springer, another edited book entitled Multi-Chaos, Fractal and Multi-Fractional Artificial Intelligence of Different Complex Systems by Elsevier in 2022, an edited book entitled Intelligent Fractal-Based Image Analysis: Applications in Pattern Recognition and Machine Vision by Elsevier and a book named Computational and Mathematical Neuroscience, Biology, & Medicine: Clinical and Medical Applications due for 2025 by Wiley-IEEE Press.
Dr. Karaca’s research interests mainly focus on complex systems sciences with applications in various terrains, applied mathematics, advanced computational methods, AI applications, computational complexity, fractional calculus, fractals and multifractals, stochastic processes, mathematical systems, different kinds of differential and difference equations, discrete mathematics, algebraic complexity, complexity science, wavelet and entropy, solutions of advanced mathematical challenges, mathematical neuroscience and biology as well as advanced data analysis in medicine and other related theoretical, computational and applied domains.